Title
Gis-Assisted Object Detection And Geospatial Localization
Abstract
Geographical Information System (GIS) databases contain information about many objects, such as traffic signals, road signs, fire hydrants, etc. in urban areas. This wealth of information can be utilized for assisting various computer vision tasks. In this paper, we propose a method for improving object detection using a set of priors acquired from GIS databases. Given a database of object locations from GIS and a query image with metadata, we compute the expected spatial location of the visible objects in the image. We also perform object detection in the query image (e.g., using DPM) and obtain a set of candidate bounding boxes for the objects. Then, we fuse the GIS priors with the potential detections to find the final object bounding boxes. To cope with various inaccuracies and practical complications, such as noisy metadata, occlusion, inaccuracies in GIS, and poor candidate detections, we formulate our fusion as a higher-order graph matching problem which we robustly solve using RANSAC. We demonstrate that this approach outperforms well established object detectors, such as DPM, with a large margin. Furthermore, we propose that the GIS objects can be used as cues for discovering the location where an image was taken. Our hypothesis is based on the idea that the objects visible in one image, along with their relative spatial location, provide distinctive cues for the geo-location. In order to estimate the geo-location based on the generic objects, we perform a search on a dense grid of locations over the covered area. We assign a score to each location based on the similarity of its GIS objects and the imperfect object detections in the image. We demonstrate that over a broad urban area of >10 square kilometers, this semantic approach can significantly narrow down the localization search space, and occasionally, even find the correct location. © 2014 Springer International Publishing.
Publication Date
1-1-2014
Publication Title
Lecture Notes in Computer Science (including subseries Lecture Notes in Artificial Intelligence and Lecture Notes in Bioinformatics)
Volume
8694 LNCS
Issue
PART 6
Number of Pages
602-617
Document Type
Article; Proceedings Paper
Personal Identifier
scopus
DOI Link
https://doi.org/10.1007/978-3-319-10599-4_39
Copyright Status
Unknown
Socpus ID
84906354756 (Scopus)
Source API URL
https://api.elsevier.com/content/abstract/scopus_id/84906354756
STARS Citation
Ardeshir, Shervin; Zamir, Amir Roshan; Torroella, Alejandro; and Shah, Mubarak, "Gis-Assisted Object Detection And Geospatial Localization" (2014). Scopus Export 2010-2014. 9221.
https://stars.library.ucf.edu/scopus2010/9221
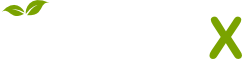
- Citations
- Citation Indexes: 42
- Patent Family Citations: 3
- Usage
- Abstract Views: 1
- Captures
- Readers: 84